Graphical Abstract
Wang, G., W. Han, S. Yuan, J. Wang, R. Y. Yin, S. Ye and F. Xie, 2024: Retrieval of high-frequency temperature profiles by FY-4A/GIIRS based on generalized ensemble learning. J. Meteor. Soc. Japan, 102.
https://doi.org/10.2151/jmsj.2024-011
Early Online Release
Graphical Abstract
Plain Language Summary: This study developed a machine learning technique to retrieve air temperature profile using hyperspectral infrared sounding data observed by a geostationary satellite with two typhoon cases. This study adopted generalized ensemble learning approach to combine three basic models: Random Forest, XGBoost, and LightGBM. The retrieved results were validated with the radiosondes and reanalysis data. As a result, the retrieval accuracy was improved enough, compared with previous studies.
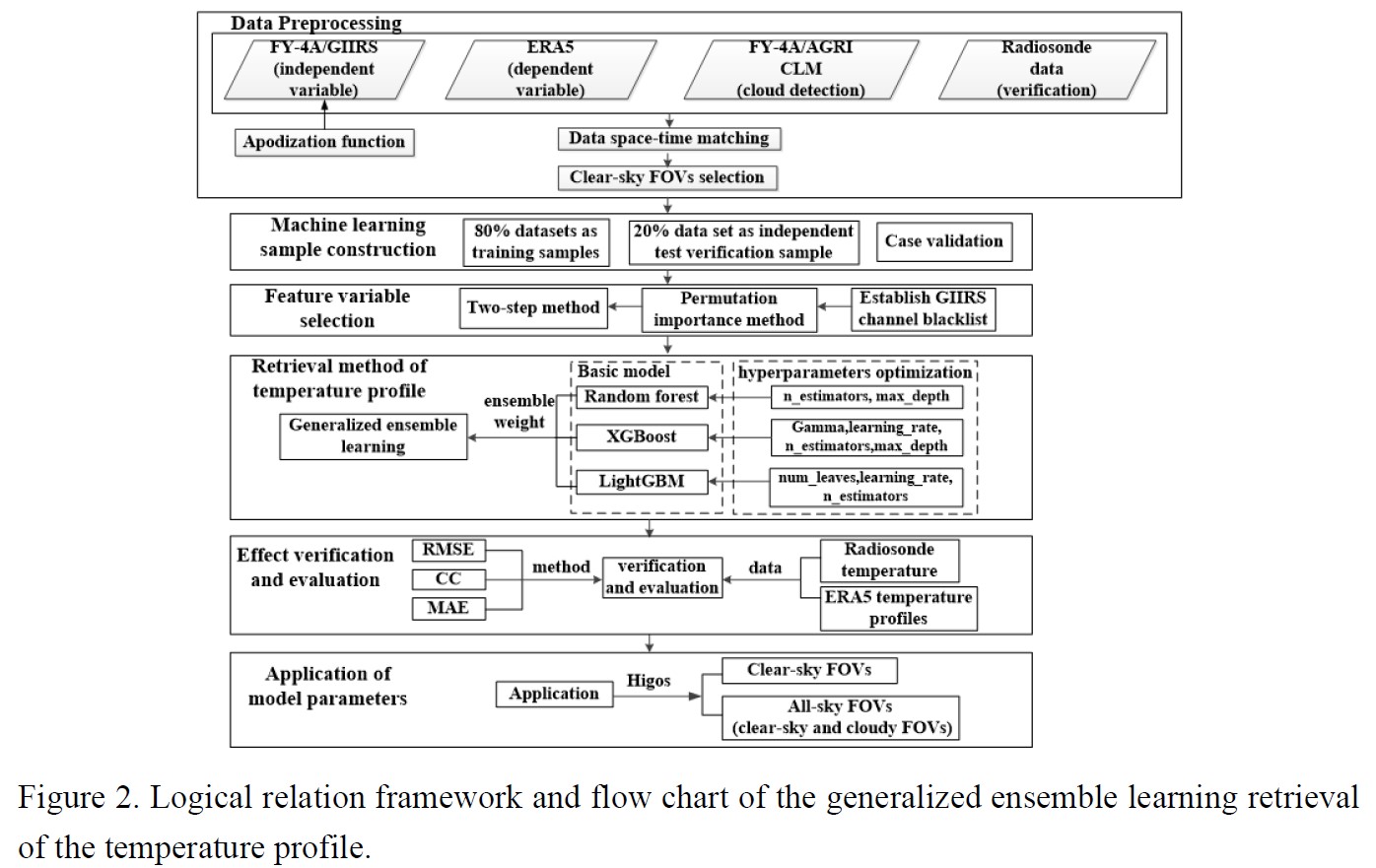
Highlights:
- A two-step feature selection method is used to select important feature variables for the FY-4A/GIIRS medium wave channel.
- Generalized ensemble learning nonlinear convex optimization is used to optimize the weight of each basic model (Random Forest, XGBoost and LightGBM).
- Under clear-sky FOVs, the RMSE of the temperature profile of generalized ensemble learning in the training dataset is less than 0.3 K, and the RMSE of the test dataset is less than 1 K between 150 hPa and 925 hPa.
- Preliminary exploration of temperature retrieval under all-sky (clear-sky and cloudy sky) FOVs also achieves high retrieval accuracy.