Graphical Abstract
Inoue, T., T. T. Sekiyama, and A. Kudo, 2024: Development of a temperature prediction method combining deep neural networks and a Kalman filter. J. Meteor. Soc. Japan, 102.
https://doi.org/10.2151/jmsj.2024-020
Early Online Release
Graphical Abstract
Plain Language Summary: This study combined the bias correction scheme of deep convolutional neural networks (CNNs) and the Japan Meteorological Agency's (JMA's) operational Kalman filter (KF) algorithm for surface temperature forecast. Verification results indicated that the proposed method outperformed both the CNN and the KF alone. Case studies showed that the CNN corrected the large horizontal structure of NWP models and the KF corrected small spatiotemporal errors.
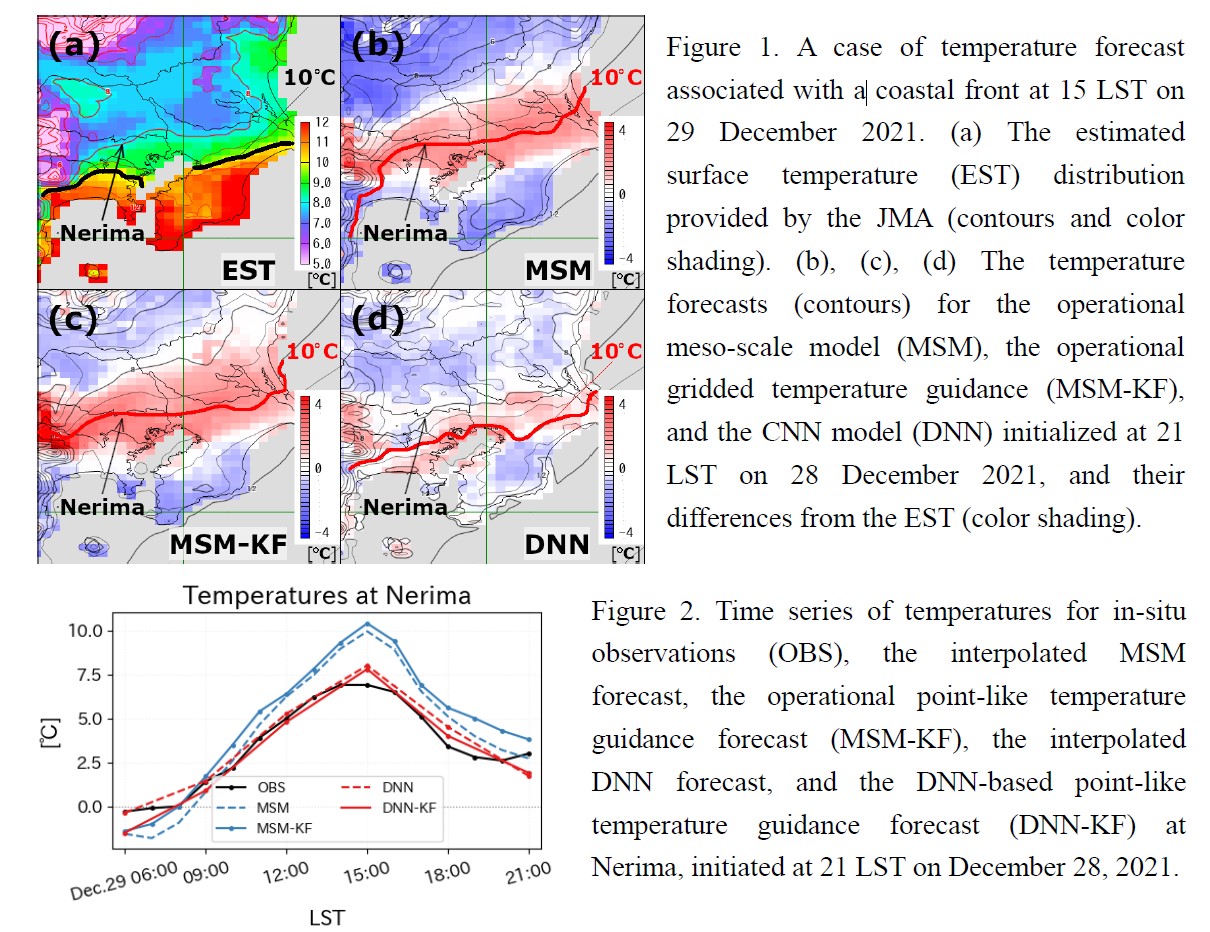
Highlights:
- This study combined the CNN-based bias correction scheme with the JMA’s operational KF algorithm.
- Verification results showed that our method outperformed both the DNN and the JMA's operational temperature guidance forecast.
- The KF has advantages of online learning that the DNN does not have. The verification demonstrated that the KF was able to follow the bias changes for NWP model updates.